SpectraLearning
Research and development on machine learning & topological computing for Shape-based classification of seismic building structural types, funded by Arup Group
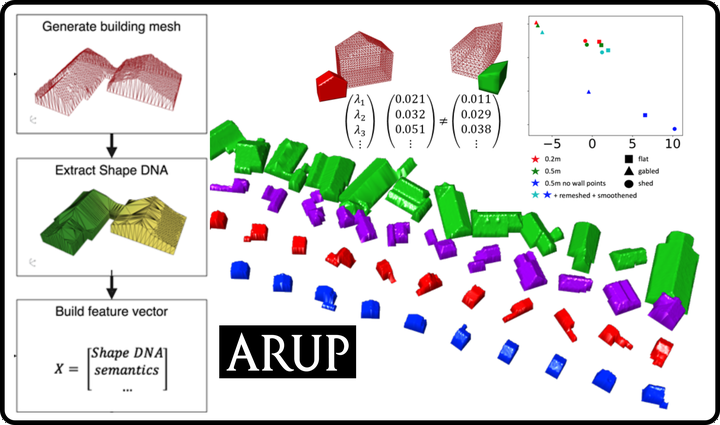
Team: Ir. R. Sulzer, Dr.ir. P. Nourian, Dr.ir. M. Palmieri, Dr.ir. J. van Gemert
Key words: Machine Learning, Shape DNA, Shape Descriptor, Pattern Recognition, Automatic Classification
In this research, our focus lies on the extraction of detailed geometric information from a point cloud gained by aerial laser scanning. We thereby follow the assumption that similar structural systems and materials can be inferred from geometric similarities. To describe the geometric shape of a building we apply Shape DNA, a spectral shape descriptor based on the eigenvalues of the Laplace-Beltrami operator. In a first experiment on a synthetically generated building stock we succeed in predicting the roof type of different buildings with accuracies above 80%, only relying on the Shape DNA. The experiment shows promising results, however, further research is necessary in order to explore the usability on real building data. In a second experiment we use a sample of buildings from the Groningen building stock. Here we can automatically predict detailed SBST information, such as the type of lateral load resisting system, with accuracies above 80% only by taking a buildings footprint area and year of construction into account.
Dissemination
Peer-Reviewed Publications:
Graduation Projects:
- Shape based classification of seismic building structural types by Raphael Sulzer
- Re-thinking the region; Systematic evaluation of residential location choice under disaster risk by Kotryna Valečkaitė